In the sprawling world of industries powered by machines and motors, the quest for effective condition-based monitoring has been relentless. The intricacies of maintaining optimal motor conditions within vast and dynamic environments have long presented a challenge. Enter the transformative solution: Effective Motor Condition-Based Monitoring, developed and scalable from Qeexo AutoML to ensure motor health. This blog delves into the innovation, technology, and impact behind this simple, yet highly effective approach to motor maintenance.
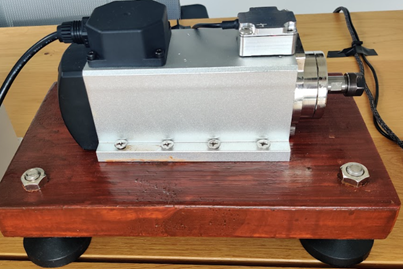
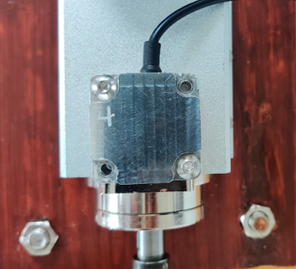
Empowering Industry with Precision
Picture an industrial setting teeming with motors driving the heartbeat of operations. Motor Condition-Based Monitoring is poised to revolutionize this landscape. Without having to take care of individual motor’s health in a large fleet by performing physical inspection, Qeexo AutoML allows us to make those motors self-aware and report anomalies as they occur with the help of the data, we train it on.
The Motor in Focus: Spindle Motor and H100 Inverter
This demo focuses on the Spindle motor, found in many industries and synonymous with CNC machines. This dynamic frequency motor is controlled by the H100 Inverter. The challenge lies in maintaining optimal conditions autonomously, a task made achievable through machine learning.
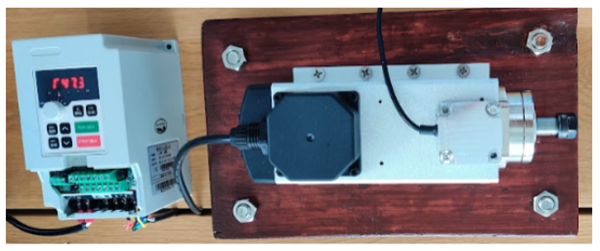
A Symphony of Data Collection and Analysis
Qeexo AutoML takes care of this very challenge, taking care of every step right from the process of data collection to model training. Vibrational data, a rich source of insights, is harnessed using the Flamenco device by TDK, featuring a high-precision accelerometer. The motor’s behavior unfolds across three states: Idle, Normal, and Fault.
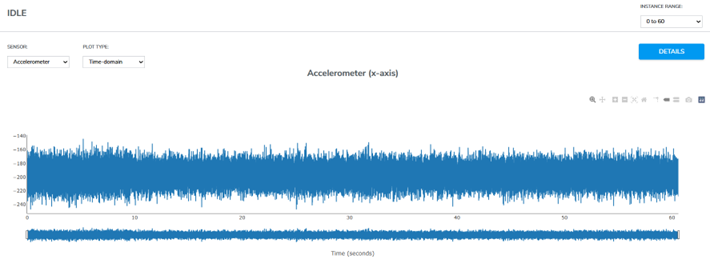
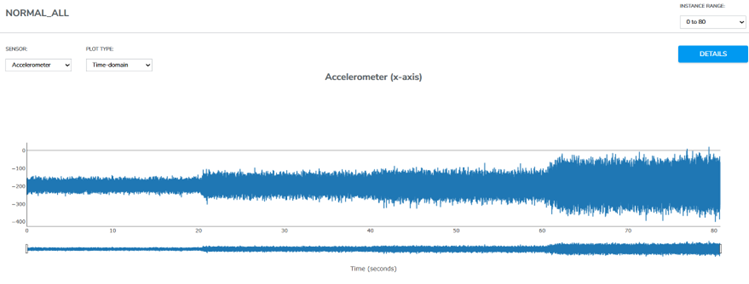
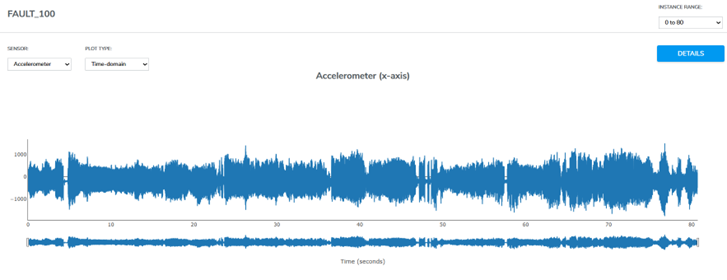
Data Collection Strategy: Crafted for Precision
The motor’s behavior is recorded across varying frequencies—25 Hz, 50 Hz, 75 Hz, and 100 Hz—enabling a comprehensive understanding of its vibrational signature. Each state is sampled, ensuring a robust dataset that mirrors real-world scenarios. According to the Nyquist theorem, the sampling rate (ODR) should be at least twice the highest frequency present in the signal to prevent aliasing and accurately reconstruct the original signal.
In my case, the motor exhibits unique features around 1kHz so I set my sampling rate to 2kHz to accurately capture the motor’s frequency.
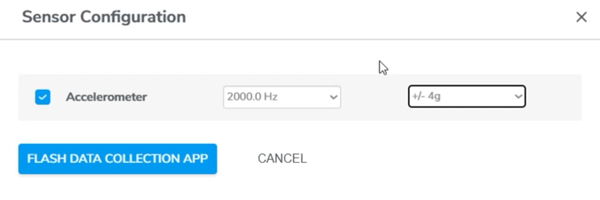
Unveiling the Fault Anomaly
To emulate a fault scenario, a simulated anomaly is introduced—a screwdriver in contact with the rotor. This friction-induced instability triggers erratic vibrations detected by the Flamenco accelerometer. The continuous contact between screwdriver and rotor ensures distinct vibrations in the “Fault” dataset, unmistakably deviating from the “Normal” class.
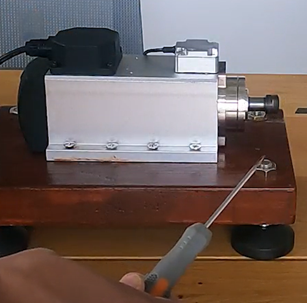
Model Training: Art Meets Science
The heart of the Motor Condition-Based Monitoring demo is the model itself. With Qeexo AutoML providing multiple models to choose from, Gradient Boosting Machine (GBM) was found to be the highest performing compared to all other models. By assigning weights to minority classes, it accommodates transitions across motor frequencies and negates misclassifications.
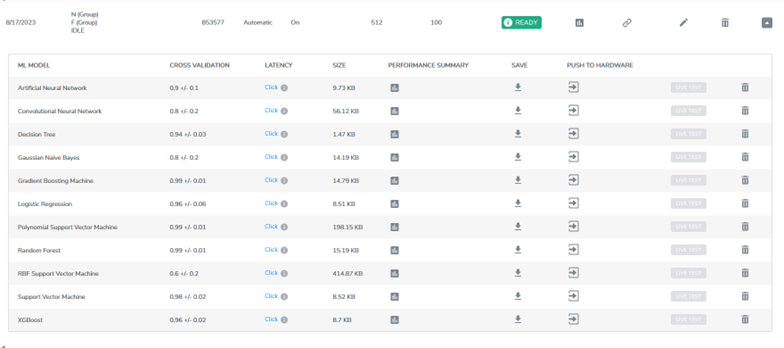
Seamless Live-Testing and Unveiling Insights
The results were—a dynamic classifier capable of distinguishing between normal, fault, and idle classes across the motor’s frequency spectrum. Swift, accurate classification changes underscore the model’s effectiveness in discerning motor health shifts in real-time.
Unlocking the Potential: Single Class Anomaly Detection
While training covers Idle, Normal, and Fault classes, there is another approach that can be taken — Anomaly Detection. By grouping Idle and Normal into a single class, the model can be trained to sense any deviation from the norm—a worn belt, loose screw, or external interference— the model flags the anomaly and alerts the technician with real-time fault detection.
Conclusion: The Era of Autonomous Motor Health
As industries continue to adopt DX strategies Motor Condition-Based Monitoring stands at the forefront of this change in thinking. In combining mechanics with sensors, this solution lays the foundation for autonomous motor health upkeep, which can be integrated into any MES, SCADA, PLC, or other manufacturing system to build predictive maintenance solutions using historical and sensor data, transforming industries into a new era where motors are no longer just mechanical entities but dynamic, self-aware systems. Welcome to the future of motor health—empowered, intelligent, and revolutionized.